- The difference between AI and ML for Fintech
- How are AI and ML used in Fintech?
- Intelligent decision making
- AI-powered chatbots
- Customer support
- Fraud detection
- Insurance management
- AI-powered financial assistants
- Predictive analytics
- Wealth management advisory
- The future of ML and AI in Fintech
- Integrate AI in Fintech with Geniusee
- Wrapping up
- AI in Fintech: FAQ
Artificial intelligence (AI) and machine learning (ML) have led the inception and rapid growth of the global financial technology or Fintech industry. Industry data suggests that by 2026, the global market size for AI in Fintech will account for an astonishing USD 26.67 billion while maintaining a CAGR of 23.17% from 2021 through 2026.
With increasing levels of process automation and digital transformation of financial services, the use cases of AI and machine learning in the finance industry are everywhere, from secured digital transactions to personalized financial advice.
Do you want to know more about machine learning and artificial intelligence in FinTech? Keep reading this article, then. We will explore some leading use cases of AI and ML in the Fintech sector. But before we do that, here is a question for you — are AI and ML the same? Let’s find out.
In this article:
The difference between AI and ML for Fintech
On the web, social media, and even in the news, you will often see that writers tend to use the terms AI and ML in Fintech interchangeably. Yet, contrary to popular belief and widespread use, those two terms do not carry the same meaning.
Simply put, machine learning is a subset under the wider domain of artificial intelligence. By using artificial intelligence, machines can practice the skill of intelligent decision-making while behaving more like humans. In contrast, machine learning expresses the skills of machines to collect new information and identify patterns without any external supervision from engineers or developers.
Are you building a digital wallet or trying to implement the right payment system? Geniusee, as a financial software development company, is ready to take your challenging project and make it rock with state-of-the-art uses of AI and ML.
Now, let’s check out some modern-day uses of AI and ML algorithms in the financial industry.
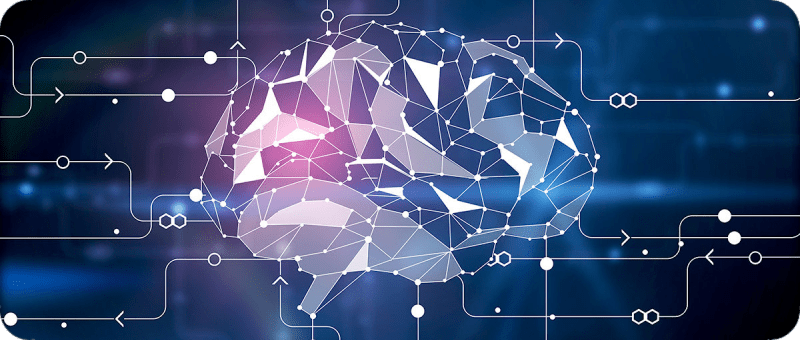
By the way...
Are you ready for AI?
IFind out if your company is ready to implement best atrificial intelligence techniques.
Tell meHow are AI and ML used in Fintech?
The Cambridge Center for Alternative Finance found that more than 90% of global Fintech companies are already relying heavily on artificial intelligence and machine learning. The statistic also shows how financial firms are leveraging AI systems. Here, we have listed a few of the uses of artificial intelligence in Fintech.
Intelligent decision making
Using AI in Fintech has enabled financial institutions to make intelligent decisions by analyzing a massive amount of data gathered in real time from national and global financial markets. The process of collection, computation, and analysis of data happens instantaneously.
Financial advisers and analysts get a clear picture of the financial industry. They can use the data to offer valuable advice on loans, insurance, and wealth management to generate higher customer satisfaction.
Instant visibility and data analysis with artificial intelligence also helps global Fintech companies make proactive decisions by anticipating financial sector trends in advance. As a result, financial institutions can propose better quotations for customers based on individual financial goals, leading to greater convenience, cost reduction, and a lower workload for all parties.
AI-powered chatbots
Artificial intelligence for Fintech has given birth to AI-driven chatbots capable of conducting detailed sentiment analysis. Call centers worldwide have to spend a bulk portion of their time and resources by answering a set of frequently asked questions from customers. It is possible to find specific patterns for customer queries and interactions through big data analytics. Fintech companies then use learning to train AI chatbots with machine learning and artificial intelligence.
As an added benefit of AI, sentiment analysis enables chatbots to answer a set of pre-programmed questions. Instead, it develops a deep understanding of customers’ relationships with financial services. In turn, it leads to a better quality of process automation, eliminating waiting time for customers. For example, when Bank of America deployed chatbots for the first time to interact with customers, the size of their customer base increased by more than a million within two months of launch.
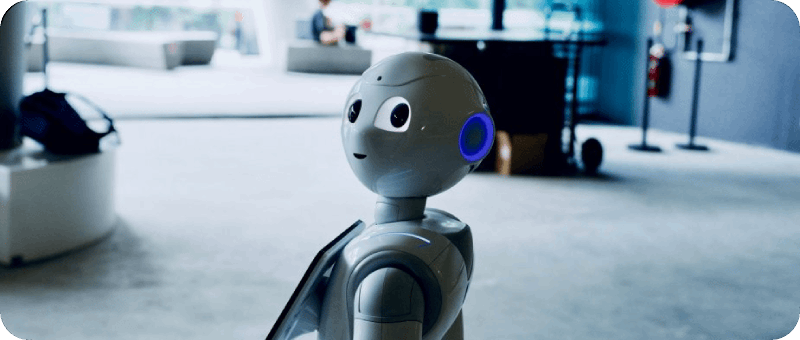
More on the topic
Virtual Assistants And Digital Custody For Banking
Financial companies need to move to a new level of service and constantly improve the service in terms of personal online customer service. This is an important competitive advantage now because it builds customer loyalty.
Find out moreCustomer support
Financial service providers can provide a superior customer experience by using AI for Fintech. The main advantage is that it consumes fewer resources to train a chatbot with artificial intelligence than to train a person. The situation carries more importance for modern-day financial institutions because governments and regulatory bodies frequently update rules and regulations related to financial services, which are difficult to follow for humans. AI technology directly addressed this shortcoming.
Industry experts estimate that banks and Fintech institutions will save 826 million work hours in 2023 by introducing automated customer support through artificial intelligence in FinTech. At the same time, customers get their queries answered within a fraction of a second, and they do not see any difference with human intelligence.
Fraud detection
The increasing use of blockchain technology has led to the popularity of smart contracts and smart wallets. However, with higher convenience for the customers, data security concerns for financial services have also multiplied. Javelin identified that financial theft accounted for 56 billion USD in 2020.
However, artificial intelligence detects fraud easily for Fintech applications by continuously keeping an eye on the entire system and all user accounts to identify and restrict any suspicious activity early.
Illegal activities such as money laundering are also a major financial security concern for financial regulators worldwide, and they spend a lot to deploy effective anti-money laundering mechanisms. ML in Fintech helps regulatory bodies detect money laundering activities quickly and take necessary measures.
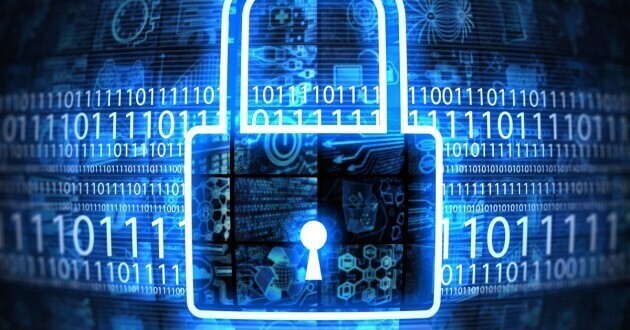
Something interesting!
Amazon Detective. Check it out!
Amazon Detective makes it easy to analyze and speedy perceive the root cause of potential protection issues or suspicious activities.
Let's seeInsurance management
By automating frequent insurance management and underwriting tasks, insurance companies can produce a greater customer experience through machine learning. Companies can also prepare detailed reports on customer profiles through credit scoring from various data points. Similarly, using AI in Fintech allows customers to access various market data, leading to more informed and data-driven decision-making for choosing an optimal insurance package.
Both the customers and the insurance providers will see a significant reduction in processing time and cost with AI-driven loan underwriting. Artificial intelligence will use data from various sources, even outside the customer’s medical records, to create links and forecasts.
AI-powered financial assistants
AI in Fintech enables the customer support team to train automated digital financial assistance for interacting with customers. The team members can use the saved time to perform more value-adding tasks for financial services. With the ability to use data analytics from internal and external sources, automated assistants also provide a wide array of information to customers that are difficult and time-consuming for humans to learn.
More interestingly, developers also utilize Natural Language Processing (NLP) for internal processing to help the digital assistants learn customers’ emotional states and facial expressions during interactions. During client onboarding, it is particularly useful because customers do not feel like they are interacting with a programmed bot; as a result, customer satisfaction is higher from the instant communications powered by speech recognition software.
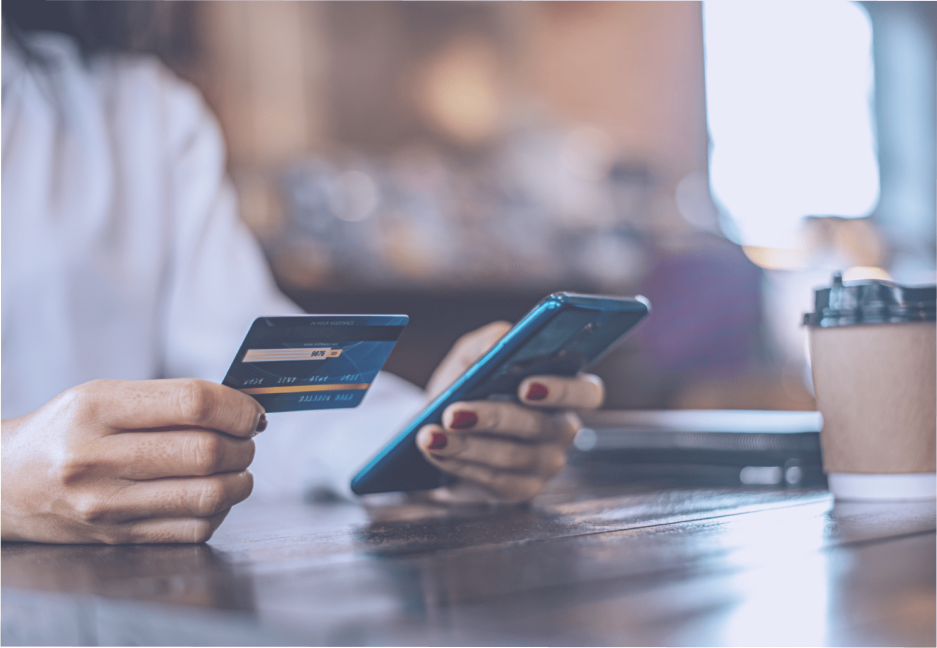
Some useful tips
Digital Banking Trends. Stay on the same wavelength with the market
Reshaped landscape of financial industry may be an obstacle, as well as opportunity. Find out more to take advantage!
Find out morePredictive analytics
Predictive analysis has revolutionized the way financial companies make decisions by integrating data science within the process. AI and ML can help companies analyze a wide array of customer and market data, streamlining the overall business development and management process. Every activity, from nurturing leads to converting revenue in financial services, takes advantage of predictive analytics.
With predictive analytics, programmers can also develop AI algorithms to compute credit scores based on the individual risk profile of the customers and offer financial services accordingly to reduce the probability of incurring bad debts in the future.
Fintech firms can also apply predictive analysis to marketing and branding campaigns. For instance, Fintech organizations can use it to proactively evaluate the effectiveness of a branding strategy on the customer's mind.
Wealth management advisory
Personalized asset and expense management advisory services are in high demand from customers. However, offering those services to lower net worth customers is not financially lucrative for Fintech companies, as they get their sales commission depending on their net worth. Fortunately, artificial intelligence and machine learning have changed the situation completely.
Financial companies can now program their banking apps to utilize AI to provide users with personalized financial advice based on spending patterns and financial conditions. This allows financial institutions to offer services to a much higher number of users without incurring the additional cost of maintaining a larger workforce. As a result, they can reduce service charges to a great extent.
More importantly, users will also benefit from significantly reduced costs, processing time, and service charges. AI-driven smart wallets can learn from users’ spending behavior and generate real-time visual statistics to support their financial advice.
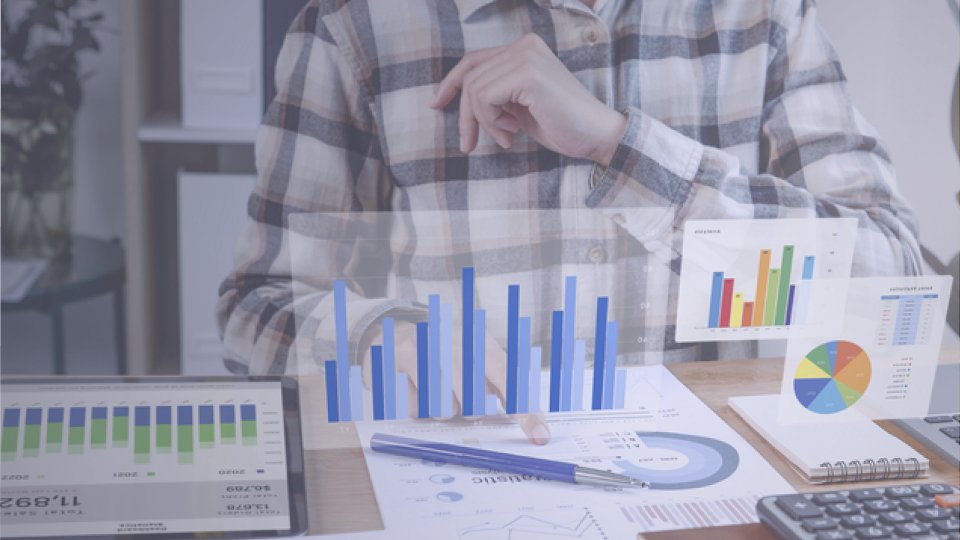
By the way...
Top Investment Management Software
Choosing the top investment management software is not an easy task. There is a lot of efficient software available, each with its own price, deployment options, and features.
See the full guideReasons for embracing AI in the Fintech
Companies are increasingly turning to artificial intelligence as a powerful tool to transform the landscape. AI's ability to analyze data arrays, automate financial processes, and make intelligent and accurate predictions has revolutionized the way financial services are delivered. Here are some compelling reasons why embracing AI in Fintech is becoming essential for staying competitive and driving innovation.
Enhanced customer experience
AI chatbots and virtual assistants enable personalized customer interactions, offering real-time support and instant responses to queries. This fosters a seamless and efficient customer experience, resulting in increased satisfaction and improved customer loyalty.
Data-driven insights
Fintech companies deal with enormous volumes of data. AI-driven analytics can swiftly analyze this data to identify patterns, trends, and valuable insights. These data-driven insights help FinTech businesses make more informed decisions, tailor offerings to individual preferences, and refine their strategies for enhanced performance.
Fraud detection and prevention
The financial sector faces significant challenges related to fraud and cyber threats AI algorithms can detect anomalous behavior and patterns, spotting potential fraudulent activities in real-time. This proactive approach helps minimize financial losses and safeguards customer trust.
Smart risk management strategies
AI-driven risk assessment models can analyze borrower data and creditworthiness more accurately and efficiently. This enables lenders to make data-backed decisions on loan approvals and minimize default rates, leading to a healthier loan portfolio.
Speed and efficiency
Artificial intelligence works as a tool for the automation of manual processes in Fintech, reducing operational bottlenecks and increasing overall efficiency. Tasks like document verification, customer onboarding, and payment processing can be streamlined, saving time and resources.
Personalized financial advice
AI algorithms can analyze customers' financial behaviors and goals to provide tailored financial advice. This personalized guidance helps customers make better financial decisions and achieve their objectives effectively.
Improved regulatory compliance
Fintech companies operate within a complex regulatory environment. AI can ensure adherence to compliance requirements by continuously monitoring transactions, detecting suspicious activities, and generating accurate reports, reducing the risk of penalties and legal issues.
Algorithmic trading
AI-powered algorithmic trading can analyze market trends, identify profitable opportunities, and execute trades with remarkable speed and precision. This allows Fintech firms to capitalize on market fluctuations and generate superior returns for investors.
Cost savings
By automating routine processes and reducing the need for manual intervention, Fintech companies can achieve significant cost savings. These cost efficiencies can be reinvested in product development and customer-focused initiatives.
Competitive advantage
Embracing AI in banking services allows companies to stay ahead of the competition. Early adopters of AI technologies can gain a competitive edge by offering innovative products, faster services, and superior customer experiences.
So AI presents companies with immense opportunities for growth, efficiency, and customer-centricity. Embracing AI-driven & ML solutions can lead to enhanced customer experiences, improved credit risk management, and strategic advantages in the fast-evolving financial landscape. As AI technologies continue to advance, Fintech firms that harness their potential will be better positioned to thrive in the digital economy.
The future of ML and AI in Fintech
AI and machine learning in Fintech not only enhance existing solutions and processes but also open up new possibilities for the banking sector. AI can create new products and services that meet the needs and expectations of customers in the era of digital transformation. Moreover, ML & AI can foster collaboration among various participants in the financial market and contribute to social and environmental well-being.
Here are some examples of how AI & ML algorithms will be used in the future Fintech sector.
Deep learning and big data in financial forecasting
AI can produce more accurate and reliable financial forecasts using big data and deep learning, analyzing various variables influencing financial markets such as economic, political, social, psychological, and others. AI can also employ neural network ML models to identify complex patterns and signals in data, which may be concealed by traditional methods.
This enables investors and traders to make more informed and profitable decisions in the market.
For example, Numerai utilizes AI to create collective intelligence from thousands of anonymous contributors who provide their predictive models based on encrypted data, using cryptocurrency Numeraire to reward top models and encourage collaboration among participants.
AI in personalized banking services
AI and ML in Fintech can utilize personalization and segmentation to create customized banking services tailored to individual customer needs and preferences. By leveraging customer data such as demographics, financial behavior, goals, and interests, AI can recommend the most suitable products and services such as loans, deposits, cards, insurances, and others. Additionally, AI can use NLP to facilitate more natural and convenient communication with customers through voice or text assistants.
For instance, Cleo employs AI to create a personalized financial assistant that helps users manage their finances via messaging apps, analyzing user transactions, providing advice on saving, investing, and budgeting, and enabling money transfers to other users.
Using AI and ML to create efficient payment and money transfer systems
AI has the potential to utilize blockchain and cryptocurrencies to establish an efficient payment and money transfer system, ensuring speed, security, transparency, and low transaction costs. AI can also employ smart contracts to automate payment and transfer execution based on predefined conditions. Biometrics and facial recognition help AI verify the identities of money senders and recipients.
For example, Circle employs AI to create a platform for exchanging money and digital assets via blockchain, using AI for risk management & analysis, fraud detection, price optimization, and improving user experience.
AI in individual investment portfolio management
AI can leverage robo-advisors to develop individual investment plans for clients that reflect their financial goals, risk tolerance, preferences, and transaction history. AI applies algorithmic approaches for automatic algorithmic trading on the market considering current situations and strategies. Additionally, AI utilizes machine learning for analyzing market movements and predicting future changes and trends in financial markets.
For example, Wealthfront uses AI to create an automated investment management service, determining the optimal portfolio for each client and conducting portfolio rebalancing, tax optimization, and automatic investing.
Using AI and ML to optimize corporate finance structures
AI can apply optimization and simulation to adjust corporate finance structures to meet various company goals and constraints such as increasing profits, reducing costs, complying with regulatory norms, risk management & control, and others.
AI analyzes company data such as revenue, expenses, assets, liabilities, capital, and others to determine the optimal balance between debt and equity, interest rates on debt, dividend strategy, and other indicators. AI can conduct scenario analysis to measure the impact of various factors on the financial reliability and sustainability of the company.
For example, American Express's product, Business Blueprint, utilizes AI to provide loans to small businesses, analyzing business data from various sources such as bank accounts, social networks, online platforms, and others to assess their creditworthiness and offer them the best lending terms.
AI for preventing financial crimes and combating money laundering
AI and ML in Fintech can utilize behavior analysis, anomaly detection, and network analysis to combat financial crimes and money laundering, which undermine trust and security in the financial industry and society as a whole.
Using transaction data, customer information, suspicious individuals and organizations, blacklists, and others, AI detects and alerts about illegal or suspicious operations. AI can also use NLP to analyze text documents such as contracts, reports, complaints, and others for fraud detection or money laundering.
For example, HSBC employs ML & AI to create a platform for preventing financial crimes, analyzing large volumes of data in real time to identify anomalous patterns and behaviors in transactions. Additionally, AI is used to generate insights and recommendations for operators and regulators to help them make quick and effective decisions on preventing financial crimes.
Integrate AI in Fintech with Geniusee
With a team of highly skilled AI developers and data scientists, Geniusee creates advanced AI-powered security solutions and algorithms that enable financial institutions to make data-driven decisions with unprecedented accuracy and speed. Our AI-driven analytics offer valuable insights into customer behavior and market trends, enabling financial technology companies to identify lucrative opportunities and make informed investment choices. As the Fintech landscape continues to evolve, Geniusee remains committed to pushing the boundaries of AI integration, helping clients stay ahead of the curve and achieve sustainable growth in this rapidly changing industry.
Explore our AI-powered app development services
Embrace artificial intelligence to automate internal processes, enhance customer engagement, and uncover valuable insights.
Wrapping Up
AI in fintech has a great range of applications for financial institutions, such as higher-quality customer support in real-time, scam prevention, insurance, asset management, and personalized financial advisory services. In addition, artificial intelligence and machine learning also contribute greatly to increasing the efficiency and accuracy of analytics, making customer interaction faster and more value-adding.
Need a professional Fintech software development team? Drop a note to Geniusee to make your project a reality.
AI in Fintech: FAQ
How to use AI in Fintech?
One of the key applications of AI in financial technology is customer experience. AI chatbots and virtual assistants are transforming the way financial services interact with customers. These intelligent bots can engage in natural language conversations, providing real-time support, and answering customer inquiries with remarkable accuracy. By automating customer support, banks, and Fintech companies can significantly reduce response times, enhance customer satisfaction, and improve overall operational efficiency.
Another significant application of AI in Fintech is in data analysis and decision-making. The financial service industry generates massive amounts of data daily, making it challenging to extract meaningful insights and identify trends manually. AI based analytics can swiftly process and analyze this data, offering valuable insights into customer behavior, market trends, and investment opportunities. With these data-driven insights, FinTech companies can make more informed decisions, develop personalized financial products, and optimize their business strategies. AI's ability to spot patterns and predict future trends allows financial institutions to mitigate risks, tailor offerings to individual needs, and achieve superior performance in a highly competitive landscape.
What are the challenges for Fintech companies in embracing AI?
Embracing AI in the Fintech industry offers numerous benefits, but it also comes with several challenges that companies need to address. Here are five main challenges.
- Data Privacy and Security Concerns. AI in Fintech heavily relies on customer data, including financial transactions and personal information. Ensuring the privacy and security of this data is paramount, as any breaches or mishandling can result in severe consequences, including loss of trust, regulatory penalties, and legal liabilities. Financial companies must invest in robust cybersecurity measures, compliance frameworks, and dataencryption to safeguard sensitive information.
- Regulatory Compliance. The financial industry is heavily regulated, and implementing AI technologies may raise additional compliance concerns. Financial regulators often require transparency in AI algorithms and may scrutinize how AI-driven decisions are made. Companies that decide to get an advantage in the use of artificial intelligence must navigate complex regulatory frameworks, ensuring that their AI solutions comply with industry standards and guidelines.
- Data Quality and Bias. AI models heavily depend on the quality and diversity of financial data used to train them. Biased or incomplete data can lead to biased outcomes and unfair treatment of certain customers. Fintech companies must be vigilant in selecting and cleaning their training data to avoid perpetuating biases and to ensure that AI systems provide equitable solutions to all users.
- Integration with Legacy Systems. Many established Fintech companies have legacy systems in place, which can be challenging to integrate with new AI technologies. Migrating from traditional systems to FinTech AI solutions requires careful planning, resource allocation, and potential redesign of existing processes. Companies must strike a balance between modernizing their infrastructure and ensuring a smooth transition without disrupting critical operations.
- Skills Gap and Talent Acquisition. The successful AI and Fintech collaboration demands a highly skilled workforce capable of developing, managing, and deploying AI solutions. There is a global shortage of AI experts, making it challenging for FinTech companies to find and retain top talent. Investing in ongoing training and talent development is crucial to building a workforce equipped with the expertise needed to drive AI initiatives effectively.
- Addressing these challenges requires a strategic approach, collaboration with regulators, continuous monitoring of Fintech and AI systems, and a commitment to upholding ethical AI practices. Overcoming these hurdles will enable FinTech companies to leverage AI's transformative potential and gain a competitive advantage in the rapidly evolving financial landscape.
What kind of AI software is used in Fintech?
Various types of AI applications in Fintech are used to cater to different needs and requirements. Here are five popular examples of AI software commonly utilized in the FinTech industry:
- Machine Learning Frameworks
- Natural Language Processing (NLP) Libraries
- Robotic Process Automation Tools
- Chatbot Development Platforms
- Data Analytics and Business Intelligence Tools
These AI software examples play a crucial role in driving innovation, improving customer experiences, and optimizing operations for Fintech companies. By leveraging these powerful financial instruments, businesses can stay ahead in a rapidly evolving industry and deliver sophisticated financial services to their clients.