The impact of predictive analytics on our daily lives is huge, with sectors such as healthcare, banking, retail, and more using data to enhance their operations. Predictive analytics uses historical data with statistics to make future decisions. Artificial Intelligence (AI) is bringing new changes and making predictive analytics models more accurate and actionable.
Data scientists believe we are entering a period where AI will oversee and manage most tasks, emphasizing its lasting presence. AI can process vast amounts of data at unprecedented speeds to help businesses optimize their workflow.
A recent report from Grand View Research further emphasizes this sentiment: “The global artificial intelligence market size was valued at USD 136.55 billion in 2022 and is projected to expand at a compound annual growth rate (CAGR) of 37.3% from 2023 to 2030.”
Let’s dive deep and examine predictive analytics and artificial intelligence and how they work together to help businesses make reliable and beneficial decisions.
In this article:
What is AI-Powered Predictive Analytics
Predictive analytics is a data-centric process that offers reliable future outcomes based on historical data and ongoing trends. It focuses on data science and how different departments within an organization can collaborate to drive profitability.
After the data mining process, the gathered data is structured and analyzed based on specific criteria and goals. However, with the advancements made in AI, large and mid-sized businesses can now use predictive analytics for processing and analyzing data more than before.
This helps understand customer preferences, pinpoint potential hazards, and devise contingency plans to minimize losses. Businesses can become more proactive instead of being reactive in making decisions.
Difference Between AI and Predictive Analytics
AI uses computers and machine learning models to analyze data and make decisions based on factors and insights. However, predictive analytics identifies trends and makes assumptions based on human intervention.
The most obvious difference is that AI is autonomous and learns continuously. In contrast, predictive analytics is often used by humans to derive decisions and solutions by analyzing current and historical datasets manually.
Don't miss out on the chance to set new standards in a tech world!
Partner with us and discover endless opportunities with AI-powered applications.
How Predictive Analytics and AI Work
AI-powered analytics harnesses machine learning based on neural networks to create valuable insights. Unlike conventional analytics, AI-based analytics primarily need human intervention during the initial phase when ML algorithms are provided with training data. Here are some applications of AI analytics.
- Demand forecasting. Leveraging its predictive capabilities, AI can anticipate product demand. By analyzing stock availability, historical purchase patterns, and seasonal trends, it can project future product needs, improving inventory management and procurement strategies.
- Data consolidation. Artificial intelligence analytics can quickly gather and analyze data from diverse platforms and systems, presenting a consolidated view. This is particularly beneficial for businesses with fragmented systems or those aiming to analyze customer information across multiple data sources.
- Business outcome projections. With the ability to process vast datasets, AI analytics can predict potential outcomes. This enhances business decision-making by providing insights into the repercussions of possible strategies.
- Audience demographic analysis. AI analytics can shed light on your audience's demographics, including their buying behaviors, locations, ages, and genders. A more detailed understanding of your audience helps you personalize content and refine customer targeting.
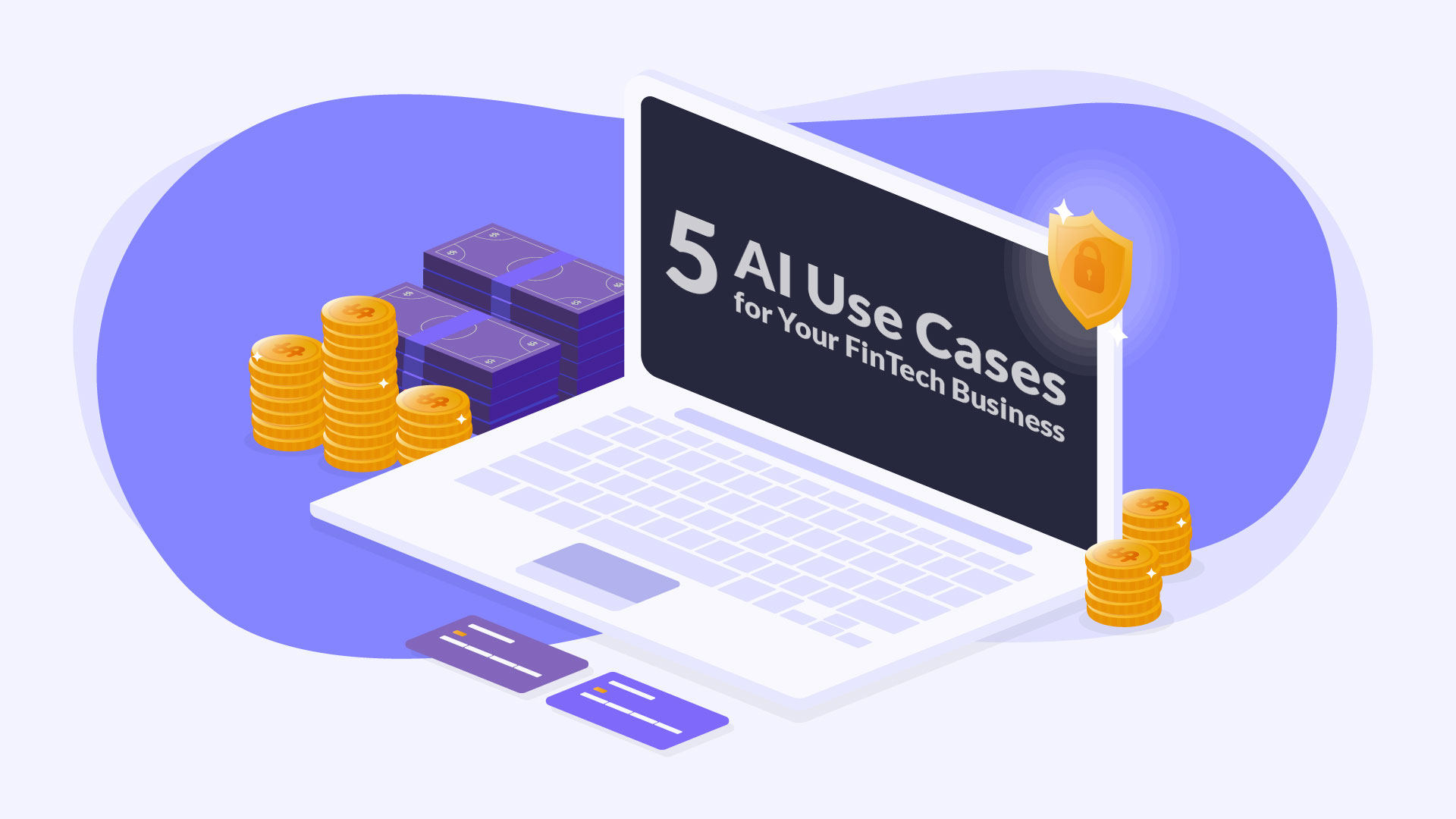
You may be interested
Top 5 Generative AI Use Cases For Your FinTech Business
Find out about FinTech operations with AI: enhanced experiences, fraud detection, personalized advice and more.
Read the articleThis equips the algorithms to recognize patterns and analyze data, generating actionable insights and forecasts from business data. Here are some applications of predictive analytics across industries.
- Retail — Predictive analytics helps retailers understand customer buying habits. By analyzing data from loyalty programs and social media feedback, retailers can forecast demand peaks, identify high-value customers, and refine their supply chain demands.
- Manufacturing — By integrating IoT and AI-powered predictive analytics, manufacturers can anticipate equipment maintenance needs. This proactive approach prevents machinery breakdowns, ensuring smooth production and easy digital transformation.
- Entertainment — Companies like Netflix exemplify the use of predictive analytics by analyzing consumer behavior. Netflix not only recommends shows but also creates content tailored to audience preferences.
- Sports — Sports organizations utilize predictive analytics for training strategies, player scouting, and injury prevention. Data-driven decisions enhance team performance and fan engagement.
- Weather forecasting — Predictive analytics enhances the accuracy of weather predictions. This is crucial for industries like agriculture and logistics, where weather conditions directly impact operations.
Any sector or business that requires predictions and analysis to run smoother works better with AI-based predictive analytics.
Thank you for Subscription!
Benefits of Using AI-Based Predictive Analytics
AI-based predictive analytics has many benefits. Its ability to expand businesses and drive growth is based on the following factors.
Enhanced decision-making. AI-driven analytics helps businesses make informed decisions by providing deeper insights from vast datasets.
Cost efficiency. AI analytics optimizes processes, leading to greater cost savings and lower resource requirements.
Personalized customer interactions. AI algorithms can tailor customer experiences based on individual preferences and behaviors, improving overall customer satisfaction.
Seamless integration. AI analytics tools can be easily integrated into existing systems, ensuring a smooth transition. A few examples of AI analytics tools are SAS Advanced Analytics, Alteryx, MicroStrategy Analytics, and TIMi Suite.
Boosted productivity. By automating data analysis and image recognition, AI allows employees to concentrate on strategic tasks, enhancing overall productivity. Real-time insights also enable quick action, driving positive business outcomes.
Advanced pattern detection. AI excels at identifying patterns within massive datasets. By leveraging the power of deep learning, businesses can detect fraud and analyze emerging trends, even when data is scattered across various platforms.
Adaptive learning. The flexibility of machine learning algorithms ensures that AI analytics tools are continuously learning. They adapt to new data, ensuring that insights remain relevant and accurate.
The Future of Predictive Analytics Using AI
AI neural networks adapt and refine their outcomes with self-learning algorithms. It excels in pattern recognition, helping you make automated decisions, freeing up time, improving inventory management, and enhancing customer service efficiency.
As AI accesses more data, its response will become more refined. Businesses harness AI-driven predictive analytics to retain and attract customers. The future of AI predictive analytics can evolve and extend to:
- Forecasting market demands and trends
- Anticipating potential risks and fraud detection
- Analyzing past and current customer behavior
- Recommending task allocations
- Crafting personalized customer experiences
Image Source: Health IT Analytics
While predictive analytics existed before AI, it was largely based on educated guesses and past sales data. Now, with AI and machine learning, predictive maintenance is more precise and comprehensive. AI systems can follow patterns overlooked by humans and offer unbiased predictions.
As businesses amass more data, their AI predictive models will improve. For example, an AI-equipped supermarket can predict a customer's needs based on their purchase history and other factors.
The emergence of AI tools like ChatGPT and platforms like TensorFlow, Scikit Learn, and Theano signals a future where predictive analytics is automated.
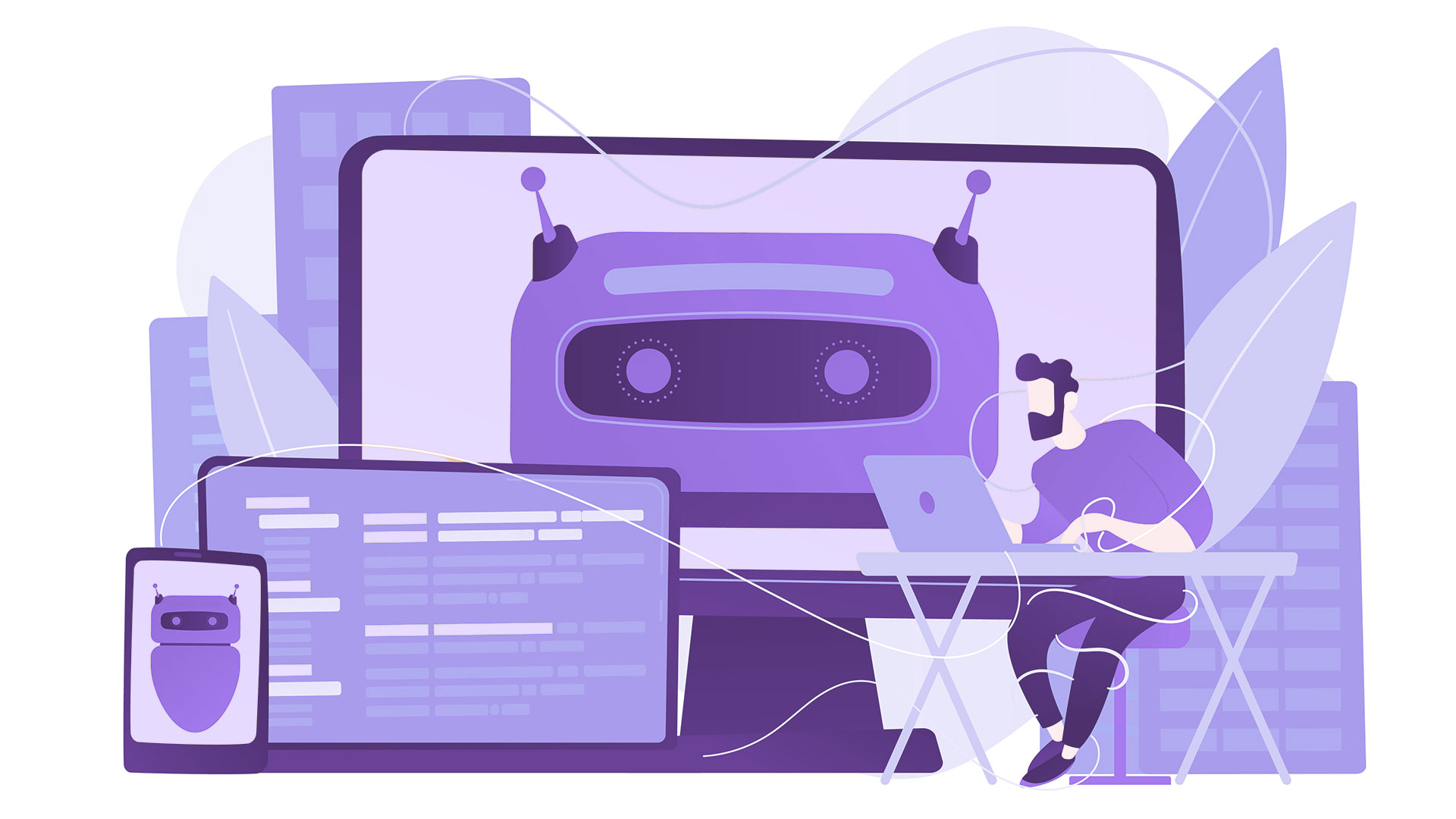
Some useful info
Comparison Of Generative AI Tools For Development And Prompt Engineering
Choose wisely: A brief overview comparing Generative AI tools.
ExploreGeniusee's Role in Software Development with Artificial Intelligence and Predictive Analytics
We at Geniusee use AI-powered apps to help your business in its digital transformation. Our expertise includes generative AI development, natural language processing, AI chatbot integration, computer vision, and AI-based predictive analytics.
So, if you need a helping hand in optimizing your business with AI and predictive analysis, consider Geniusee. As experts in software development and AI integration, we offer dedicated services that can help your business analyze and optimize with real-world data.
Wrapping Up
As datasets expand, the integration of AI in predictive analytics is also expected to increase. Merging big data with AI-driven analytics offers many advantages, improving the data analysis process and the overall business performance.
Companies using AI or aiming to adopt AI-powered predictive analytics should take essential steps in updating their systems, adjusting their risk strategies, and minimizing potential user mistakes. While this is easier said than done, Geniusee can help your business stand out with the best data analytics and decision-making algorithms.
So, carefully weigh your options and adopt the best AI-based predictive analytics for your existing operational structure and business.