- What is MLOps?
- How MLOps Works?
- What Problems Does MLOps Solve?
- How does MLOps differ from DevOps and DataOps?
- Main Benefits of MLOps Solutions
- MLOps Risks & Challenges
- Best MLOps Practices
- How to implement MLOps?
- The Future of MLOps Infrastructure
- Geniusee Experience in How to Build MLOps Solutions
- Conclusion
Machine Learning Operations (MLOps) is a set of practices that includes Machine Learning, DevOps and Data Engineering elements. The main aim of the union is reliable and efficient deployment and maintenance of Machine Learning systems in production.
In other words, it is a way to turn machine learning methods and technologies into a useful tool for solving business problems.
It is important to understand that the production chain begins long before the development of the model. Its first step is to define a business problem, a hypothesis about the value that can be extracted from the data, and a business idea for applying it.
In this article, we will review MLOps best practises, MLOps Risks & Challenges and MLOps pros and cons that automate and shorten the machine learning cycle.
In this post:
What is MLOps?
MLOps (combining machine learning technologies and processes and approaches to implementing the developed models in business processes) is a new way of cooperation between business representatives, scientists, mathematicians, machine learning specialists and IT engineers in creating artificial intelligence systems.
MLOps is a culture and set of practices for integrated and automated lifecycle management of machine learning systems, combining their development and operations maintenance, which includes integration, testing, release, deployment and infrastructure management. We can say that MLOps solution extends the CRISP-DM methodology with the help of an Agile approach and technical tools for automated operations with data, ML models, code and environment. Putting MLOps into practice is expected to help avoid common pitfalls and problems faced by Data Scientists working in accordance with the classic phases of CRISP-DM. We will also talk about other advantages that this concept gives to business further.
The very concept of MLOps arose as an analogy to the concept of DevOps in relation to models and technologies of machine learning. DevOps is an approach to software development that allows you to increase the speed of implementation of individual changes while maintaining flexibility and reliability using a number of approaches. These approaches include continuous development, dividing functions into a number of independent microservices, automated testing and deployment of individual changes, global health monitoring, rapid response system detected failures, etc.
DevOps defined the software lifecycle, and the idea came up in the community to use the same technique for big data. DataOps is an attempt to adapt and expand the methodology taking into account the peculiarities of storing, transferring and processing large amounts of data in a variety of and interacting with each other platforms.
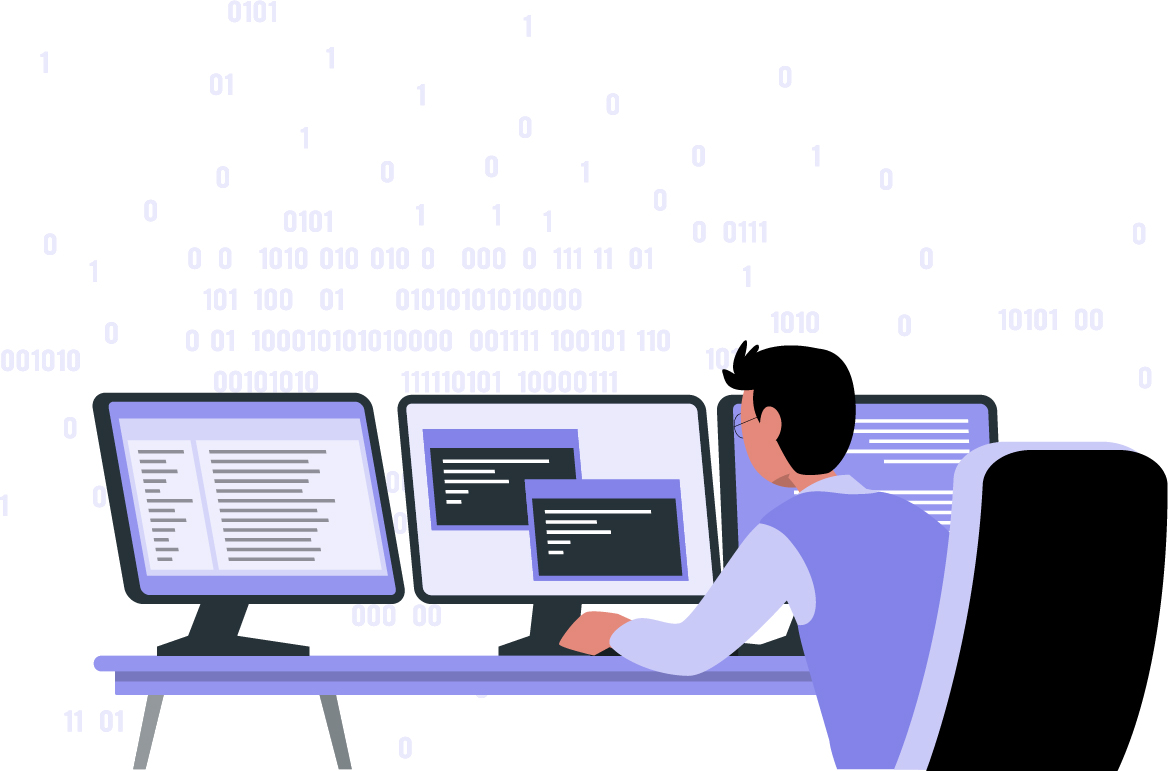
Knowledge transfer
What is DevOps?
Still guessing why everyone buzzes about DevOps and its importance? Don’t waste a chance to find out why - the answer is in this article.
Learn itWith the emergence of a certain critical mass of machine learning models implemented in business processes of enterprises, a strong similarity between the life cycle of mathematical machine learning models and the software life cycle was noticed. The only difference is that the algorithms of the models are created using MLOps tools and methods. Therefore, the idea naturally arose to apply and adapt already known approaches to software development for machine learning models. Thus, the following key stages can be distinguished in the life cycle of machine learning models:
- defining a business idea;
- training the model;
- testing and implementation of the model in the business process;
- operation of the model.
When it becomes necessary to change or retrain the model on new data in the process of operation, the cycle is restarted - the model is refined, tested, and a new version is deployed.
In this life cycle, it seems logical to use DevOps tools: automated testing, deployment and monitoring, formalizing the calculation of models in the form of separate microservices. But there are also a number of features that prevent the direct use of these tools without additional ML strapping.
How MLOps Works?
MLOps is about teamwork and interaction between different IT teams. And how should these different teams in the same company interact? And who should run this MLOps process?
MLOps is a set of best practices, but there is no universal MLOps; in each case, the approach will be different. Therefore, the work begins with the Data Science team - a kind of "artists" who come up with an ML approach to solving the problem, which algorithms to use, how to group, train, how to measure their quality, etc. The output is, for example, Jupyter Notebook. It can play the role of good documentation with description, structure, visualization, examples and gives a clear understanding to the whole team of how self-learning algorithms solve a business problem.
Next, the ML engineer goes to Data engineers, DevOps and agrees on how to turn the laptop into an understandable ML pipeline, automated, versioned, scalable, including feedback, monitoring, and reporting processes. Each specialist can close some part of themselves, for example, using Spark at the data preparation stage. The system engineer will deploy Kubeflow to automatically start data training processes, rolling out new versions of these algorithms. Developers will figure out how to integrate the results of this model into the platform, whether it will be unloading predictions or whether it will be a service that will contain the model inside and return some kind of ML result upon request.
A complex picture of the interaction of everything with everyone. But when a framework is already built around the product, the ML pipeline is automated, monitoring, integration, communication becomes easier, somewhere it can be fully automated so that the Data Scientist will no longer be ruled by an abstract laptop, but some parts of the automated pipeline already in DSL this ML pipeline.
On projects, different people from different teams try to take on this role. Some Data Scientists are engaged in ML engineering. Sometimes Data Engineers, DevOps or Systems Engineers drive this process. But each of them looks at the situation with its own specifics, so an independent arbitrator is needed to resolve the differences.
Therefore, a separate role arose - an ML engineer. It is needed so that there is a person who would take responsibility for building automation from start to finish. Accordingly, it is in his interests to agree with Data-, software-, system engineers, and Data Scientist - so that what they are responsible for happens.
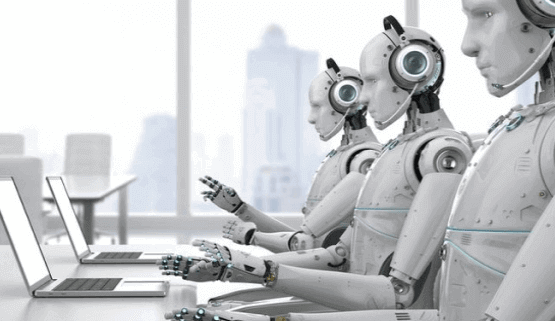
Interesting practices
Top 5 test automation tips
Automation is new black. All industries are benefiting from simplifying processes and workflow. Do you want to become one of them?
Check it outWhat Problems Does MLOps Solve?
Managing such systems on a large scale is not an easy task. There are many bottlenecks that need to be taken into account. The following are the main problems that teams face and that MLOps helps to solve:
- Lack of data scientists capable of developing and deploying scalable web applications. A new profile of machine learning engineers is emerging in the market these days to address this need. Their business stands at the intersection of data science and DevOps.
- Change business goals in the model. With many dependencies on ever-changing data, the need to maintain model performance standards, and to provide AI-driven control, retraining a model in response to changes in business goals is no easy task.
- Mutual misunderstandings between technical departments and business teams, which find it rather difficult to find a common language within the framework of joint work. Most often, it is this misunderstanding that causes the failure of large projects.
- Risk assessment. The nature of the “black box” of such machine learning and deep learning systems is a matter of constant debate. Models tend to deviate from what they were originally intended for. Assessing the risk/cost of such deviations is a very important and thorough step.
For example, the price of an inaccurate YouTube video recommendation will be significantly lower than the cost of flagging an innocent person as a scammer and then blocking their account and rejecting their loan applications.
How does MLOps differ from DevOps and DataOps?
By analogy with DevOps and DataOps, business has a need to organize continuous cooperation and interaction between all participants in the processes of working with machine learning models from business to engineers and Big Data developers, including Data Scientists and ML specialists. This business need is connected with the popularization of Machine Learning methods and the growth of their practical implementations.
The concept of MLOps is still quite young. For the first time, the professional community spoke publicly about the need for integrated ML lifecycle management in industrial operation (production) around 2018, after one of the Google presentations. Despite its relatively young age, the demand for MLOps is getting bigger and bigger every day.
In practice, the problem of introducing ML-models into a real business is not limited to data preparation, development and training of a neural network or other Machine Learning algorithm. The quality of a production solution is influenced by many factors, from verification of datasets to testing and deployment in a production environment in the form of a reliable Big Data application. This means that the actual results of prediction or classification depend not only on the neural network architecture and the machine learning method proposed by Data Scientist, but also on how the development team has implemented this model, and the administrators have deployed it in a clustered environment.
Also, the quality of the input data (Data Quality), sources, channels and the frequency of their receipt are important, which belongs to the area of responsibility of the data engineer. Organizational and technical obstacles in the interaction of multidisciplinary specialists involved in the development, testing, deployment and support of ML solutions lead to an increase in the time required to create a product and a decrease in its value for the business. To remove such barriers, the concept of MLOps was invented, which, like DevOps and DataOps, seeks to increase automation and improve the quality of industrial ML solutions, paying attention to regulatory requirements and business benefits.
Main Benefits of MLOps Solutions
MLOps solution, or DevOps for machine learning, makes collaboration possible not only for data teams, but also for analysis operations professionals and IT engineers. It also increases the speed of model development and its deployment with the help of monitoring, validation and management systems for machine learning models.
Creation of reproducible workflows and models.
The main MLOps benefits allow you to:
- reduce variation in model iterations and provide resiliency for enterprise-level scenarios with reproducible learning and models;
- use dataset registries and advanced model registries to track resources;
- provide improved traceability by tracking code, data, and metrics in the execution log;
- сreate machine learning pipelines to design, deploy, and administer reproducible model workflows for consistent model delivery.
Easy deployment of high precision models in any location.
With the help of MLOPs solution you can:
- deploy high precision models quickly and confidently;
- use automatic scaling, managed clusters of CPUs and GPUs with distributed learning in the cloud;
- pack models quickly, ensuring high quality at every step through the use of profiling and model validation;
- use managed deployment to migrate models to the production environment.
Effective management of the entire machine learning life cycle.
MLOPs benefits enable you to:
- use the built-in integration with Azure DevOps and GitHub actions to plan, automate, and manage workflows efficiently;
- streamline model training and model deployment pipelines, use continuous integration / continuous delivery to simplify retraining, and integrate machine learning easily into existing release processes;
- use advanced data bias analysis to improve model performance over time.
Machine Learning Resource Management System and Control.
MLOPs benefits also help you to:
- keep track of version history and model origin to enable auditing;
- evaluate the importance of features and create more advanced models with minimal bias using uniform distribution metrics;
- set calculation quotas for resources and enforce policies to ensure compliance with security, privacy, and compliance standards;
- create audit trails to meet regulatory requirements as you mark machine learning resources and automatically trace experiments.
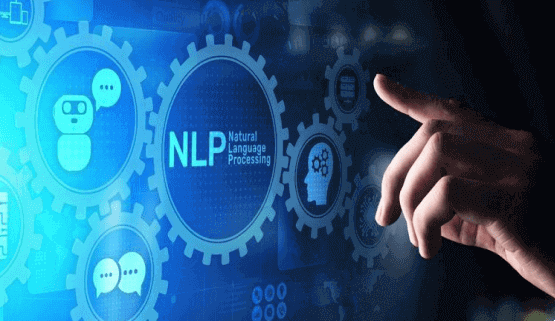
Wait, what?
What is Neuro-Linguistic Programming?
Do you know how to use AI and ML to learn your computer analyze text instead of you? Structure texts, extract insights? No magic outside of Hogwarts, only NLP.
AlohomoraMLOps Risks & Challenges
A comprehensive answer to the fundamental question of business about how applicable ML-models are for solving problems and the general question of AI and MLOps adoption are key challenges in the development and implementation of MLOps approaches. Initially, one of the biggest MLOps disadvantages is that businesses are skeptical about introducing machine learning into processes as it is difficult to rely on models in places where people used to work in the past. For business, programs appear to be a "black box", the relevance of the answers to which has yet to be proven. Besides, in certain spheres, for example, in banking, telecom operators business and others, there are stringent requirements of state regulators. All systems and algorithms that are implemented in banking processes are audited. To solve this problem, to prove to business and regulators the validity and correctness of the responses of artificial intelligence, monitoring tools are introduced along with the model. In addition, there is an independent validation procedure required for regulatory models that meets the requirements of the Central Bank. An independent expert group audits the results obtained by the model, taking into account the input data.
The second challenge is the assessment and consideration of model risks when implementing a machine learning model. A well-known internet debate about whether the dress was white or blue showed that there might be cases when even people cannot answer a question with absolute certainty. Then it would only be natural that artificial intelligence also should have the right to make a mistake. It is also worth considering that data can change over time, and models need to be trained to produce a sufficiently accurate result. So that the business process does not suffer, it is necessary to manage model risks and monitor the model's performance metrics, regularly retraining it on new data.
But after the first stage of mistrust, the opposite effect begins to appear. The more models are successfully introduced into processes, the more the business grows an appetite for the use of artificial intelligence - more and more new problems are found that can be solved by machine learning methods. Each task launches a whole process that requires certain competencies:
- data engineers prepare and process data;
- data scientists use machine learning tools and develop a model;
- IT implements the model into the system;
- ML engineers determine how to correctly integrate this model into the process, which IT tools to use depending on the requirements for the mode of application of the model, taking into account the flow of calls, response time, etc.
- ML architect designs how a software product can be physically implemented in an industrial system.
The whole cycle requires a large number of highly qualified specialists. At a certain point of development and the degree of penetration of ML-models into business processes, it turns out that it becomes expensive and inefficient to scale the number of specialists linearly in proportion to the increase in the number of tasks. Therefore, the question about “How to automate and launch an MLOps process?” arises - the definition of several standard classes of machine learning problems, the development of standard data processing pipelines and additional training of models. In the ideal picture, solving such problems requires professionals who are equally well versed in competencies at the intersection of BigData, Data Science, DevOps and IT. Therefore, the biggest problem in the data science industry and the biggest challenge in organizing MLOps processes is the lack of such competence in the existing training market. Specialists who meet such requirements are currently unique in the labor market and are worth their weight in gold.
Best MLOps Practices
1. ML Pipelines
One of the main concepts of Data Engineering is the data pipeline. A data pipeline is a cycle of transformations that are applied to data between its source and an end destination. They are usually explained as a graph in which each node is a transformation and edges represent dependencies or execution order.
2. Hybrid Teams
We’ve already established that in order to be successful we need a mixed team that has that skill set. Most likely it would consist of a Data Scientist (ML Engineer), a Data Engineer and a DevOps Engineer.
It is important to understand that a Data Scientist alone can’t achieve the goals of MLOps.
3. Model and Data Versioning
In a traditional software world you need only versioning code, because all behavior is determined by it. In ML code is a little different. In addition to the familiar versioning code we also need to track model versions, the data used to train it, and some meta-information like training hyperparameters.
4. Model validation
ML models are harder to test than DevOps one, because no model gives absolutely accurate results. This means that model validation tests need to be surely statistical in nature, rather than having a binary pass/fail status.
It’s also not enough to track one metric for the entirety of the validation set.
5. Data validation
A good data pipeline usually starts by validating the input data. In addition to basic validations that any data pipeline performs, ML pipelines need higher level validation statistical properties of the input. For example, if the average diversion of a feature changes considerably from one training dataset to another, it is likely to affect the trained model and model predictions.
6. Monitoring
For ML systems, monitoring becomes even more important than monitoring production systems. It is because their performance depends not just on factors that we have some control over, like infrastructure and our own software, but also on data, over which we have less control. Therefore, in addition to monitoring standard metrics like latency, traffic, errors and saturation, we also need to monitor model prediction performance.
How to implement MLOps?
Today, many IT integrators and vendors of Big Data systems offer comprehensive MLOps solutions based on open technologies with pre-project survey, implementation and support services. The choice, as usual, is up to the customer. However, no matter how the company implements the Machine Learning Operations approach, it will include the following steps:
- Determining the level of MLOps-maturity in the enterprise;
- Organizational changes in corporate culture, business processes and structures (functional groups and project teams);
- Design and implement automated ML training and maintenance pipelines using appropriate technical tools.
You can find out how to do this in practice by introducing big data technologies and machine learning into private business digitization projects by contacting the Geniusee team.
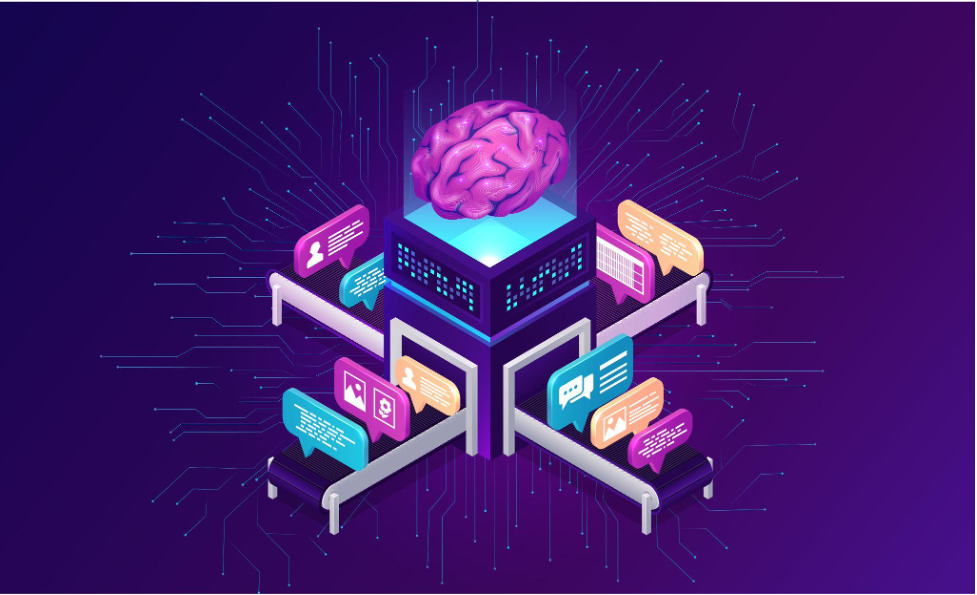
Rise of the machines or still not?
Deep learning for Computer Vision
Computer vision is very similar to human vision, only superior. It can inspect and analyze thousands of products or processes in a matter of minutes, unlike the human brain.
More info on the issueThe Future of MLOps Infrastructure
MLOps is a growing area that lacks competencies and will gain momentum in the future. In the meantime, it is advisable that the best practices and DevOps practices should be employed. The main goal of MLOps is to use ML models more efficiently to solve business problems. But this raises many questions:
- How to shorten the time for launching models into production?
- How to reduce bureaucratic friction between teams of different competencies and increase the focus on collaboration?
- How can you track models, manage versions, and organize effective monitoring?
- How to create a truly cyclical life cycle for a modern ML model?
- How to standardize the machine learning process?
The answers to these questions will largely determine how quickly MLOps will reach its full potential.
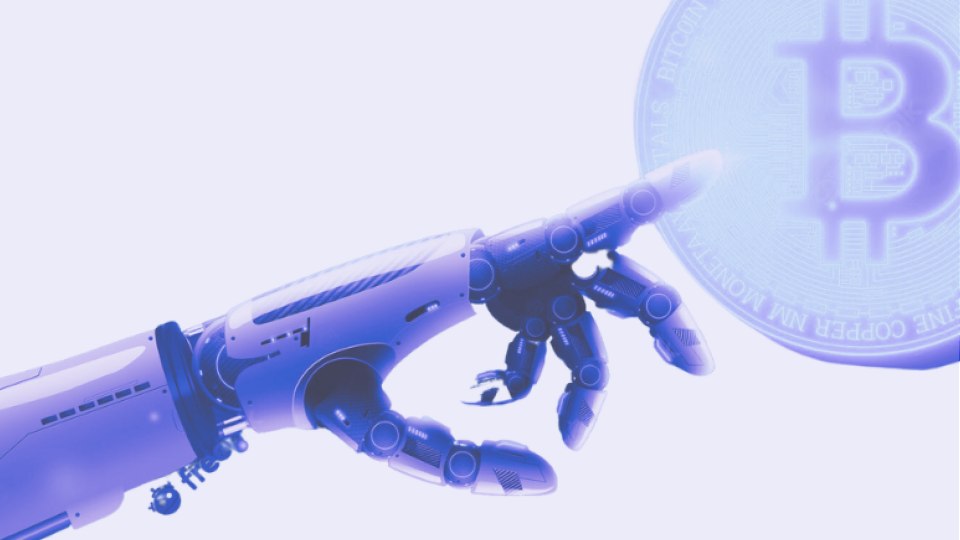
Estimate yourself
Is your business ready to use AI?
AI is across industries trend that reshaping landscapes for many markets. Still you can’t just wake up one day and implement it. See why.
Show meGeniusee Experience in How to Build MLOps Solutions
We help our clients turn their AI prototype into a real-world application or scale it to process more users. We offer MLOps services that take the pain and help bring the model to production, and launch products and updates faster and with more control.
Based on our experience, we can define the most significant Agile approaches pros of MLOps to build a MLOps solution in relation to the specifics of industrial Machine Learning deployment:
- Shorter lead times for quality results through reliable and efficient machine learning lifecycle management;
- reproducible workflows and models thanks to Continuous Development / Integration / Training (CI / CD / CT) methods and tools;
- easy deployment of high-precision ML models anywhere and anytime;
- system of integrated management and continuous monitoring of machine learning resources;
- elimination of organizational barriers and pooling the experience of multidisciplinary ML-specialists.
Thus, speaking about pros of MLOps, another great plus is that the following aspects of ML operations can be optimized using MLOps:
- unify the release cycle of machine learning models and software products created on their basis;
- automate testing of Machine Learning artifacts, such as data validation, testing of the ML model itself and its integration into a production solution;
- implement agile principles in ML projects;
- support machine learning models and datasets for them in CI / CD / CT systems;
- reduce technical debt on ML-models.
As ML matures from research to applied business solutions, we need to improve the understanding of its operational processes.
Conclusion
The following table summarizes MLOps’ main practices and how they relate to DevOps and Data Engineering practices: