The global LLM market is projected to grow from $1,590 million in 2023 to $259,8 million in 2030. During the 2023-2030 period, the CAGR will be at 79,80%.
As AI and natural language processing technologies continue to evolve, large language models are emerging as powerful tools for businesses seeking to streamline operations, enhance customer experiences, and gain valuable insights.
In this article, we will explore six promising use cases for LLM where these large neural network models can enable automation, personalization, and data analysis across an organization. From chatbots to predictive analytics, large language models are poised to transform key business functions. Read on to learn how your company can leverage these systems today to work smarter, drive innovation, and plan for the future.
In this article:
Understanding large language models (LLMs)
What are LLMs?
Large language models (LLMs) are AI systems that have been trained on massive amounts of data to understand and generate language. They are able to understand the relationships between words and use that understanding to generate fluent text. LLMs power many AI technologies today in LLM use cases like chatbots, machine translation, and automated writing.
How do LLMs work?
LLMs are trained using a technique called self-supervised learning. They are exposed to huge datasets of text from which they learn to understand language by predicting the next word in a sequence. Over time, they get better at predicting the right next word, which enables them to generate coherent text. The models can then apply this learned knowledge of language to LLM use cases like translation, summarization, and dialogue.
Applications in business
In 2025, 50% of digital work is estimated to be automated through apps using these language models. LLMs have many practical business applications. Use cases for LLMs include automating customer service interactions through chatbots, generating product descriptions and marketing copy, translating content into different languages, and more.
With proper oversight and governance, LLM business use cases can be implemented responsibly to benefit both companies and their customers.
6 use cases of LLM in business
Customer service chatbots
As businesses work to improve customer experiences, chatbots powered by large language models offer an efficient way to handle basic inquiries and requests.
Automated response chatbots can respond immediately to common questions or concerns, providing customers with quick answers and information. By analyzing frequently asked questions and typical conversations, chatbots learn to understand common customer requests and provide appropriate responses.
This automation allows human customer service agents to focus on more complex issues.
Real-world example: Delta Airlines
Known for prioritizing customer experience, Delta uses LLMs in their Ask Delta chatbot.
This AI-powered assistant helps customers with tasks like flight check-in, tracking luggage, and finding flights. The LLM's ability to understand natural language allows for smooth interaction and quick resolution to inquiries, leading to a reported 20% decrease in call center volume for Delta.
Thank you for Subscription!
Content creation and marketing
Large language models can generate hyper-personalized content for your audience at scale by leveraging user data to tailor messaging to individual interests and needs.
Manually curating content is time-consuming and difficult to scale. LLMs can help in different large language model use cases, such as suggesting related articles, summarizing key points, and rewriting or reorganizing content to resonate better with your audience.
They extract insights from large datasets that would be impossible for humans to analyze using LLMs in a reasonable time frame. By combining content from various sources, LLMs create a more complete picture of topics that matter to your customers.
Real-world example: Washington Post
This esteemed publication uses an LLM called Heliograf to automate specific aspects of content creation. Heliograf assists journalists in significant language model use cases such as generating drafts, suggesting headlines, and surfacing relevant information, ultimately accelerating the news writing process without compromising quality.
Market research and sentiment analysis
Market researchers use LLMs to analyze consumer sentiments and opinions. By processing large volumes of data from social media, reviews, surveys, and online forums, LLM can determine overall perceptions of brands, products, and services.
LLM tracks discussions across social networks to gain insights into audiences' attitudes. For example, they can analyze Facebook posts and comments, tweets, blog posts, and other social data to gauge how people feel about a new product launch or marketing campaign. LLM identifies critical themes and trends and gains a broad understanding of the prevailing mood.
Real-world example: Amazon
Amazon employs LLM-based sentiment analysis to analyze product reviews, helping them understand customer satisfaction and improve product offerings.
Document classification
LLMs can also categorize and organize documents by topic, sentiment, or other attributes. They are fed thousands of examples of classified documents during training to learn the linguistic and semantic patterns associated with each category.
Businesses can leverage classification LLMs use cases to automatically sort and filter documents, allowing employees to find the information they need more quickly and easily. Classification also enables more advanced text analytics, such as identifying trends in customer feedback or spotting anomalies.
Real-world LLM use case: JPMorgan Chase
This major financial institution leverages LLMs to categorize various documents, including loan applications, financial statements, and customer correspondence.
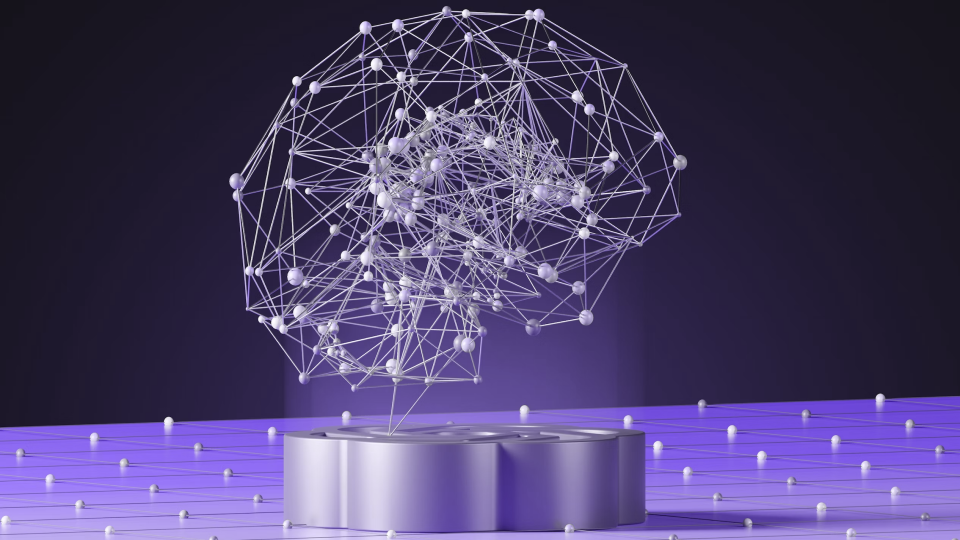
More on the topic
NLP vs LLMs vs deep learning models
Are you considering using artificial intelligence technologies in your business? Learn what is behind this tech in our article.
Read moreVirtual assistants
Large language models (LLMs) power many virtual assistants and productivity tools by enabling natural language processing (NLP). NLP allows these systems to understand complex human language input, whether through speech, text, or some other format.
With NLP, virtual assistants can handle various routine tasks like scheduling meetings, managing to-do lists, setting reminders, and more.
Real-world LLM use case: Samsung (Bixby)
Samsung integrates LLMs into their Bixby virtual assistant. Bixby can understand natural language, allowing users to control their Samsung devices, get weather updates, translate languages, and more.
Translation and localization
When translating or localizing content, there are several use cases of LLMs:
LLMs can use their massive datasets to suggest contextually appropriate translations for phrases and terms. They have been trained on huge amounts of data, so they understand nuances that machine translation alone may miss.
LLMs can also suggest synonyms, related phrases, and semantically similar terms for key words and phrases to help human translators. This aids localization by proposing regionally appropriate alternatives. For example, an LLM may suggest ''lorry'' instead of ''truck'' for UK English.
Real-world example: Airbnb
Airbnb uses LLMs to translate their platform content, including listings, descriptions, and reviews, for travelers worldwide.
Conclusion
In summary, large language models present exciting new capabilities for businesses in customer service, content creation, data analysis, research, and more.
As LLM applications continue to advance, they will enable organizations to work more quickly and efficiently. However, businesses must thoughtfully evaluate the ethics, risks, and best practices before implementation.
Overall, LLM development services have the potential to transform operations but require diligent planning to realize the benefits while proactively addressing the challenges. With careful consideration, companies can harness these powerful tools to enhance productivity and provide innovative solutions.